Spatial data in the cloud – part 2, NoSQL databases
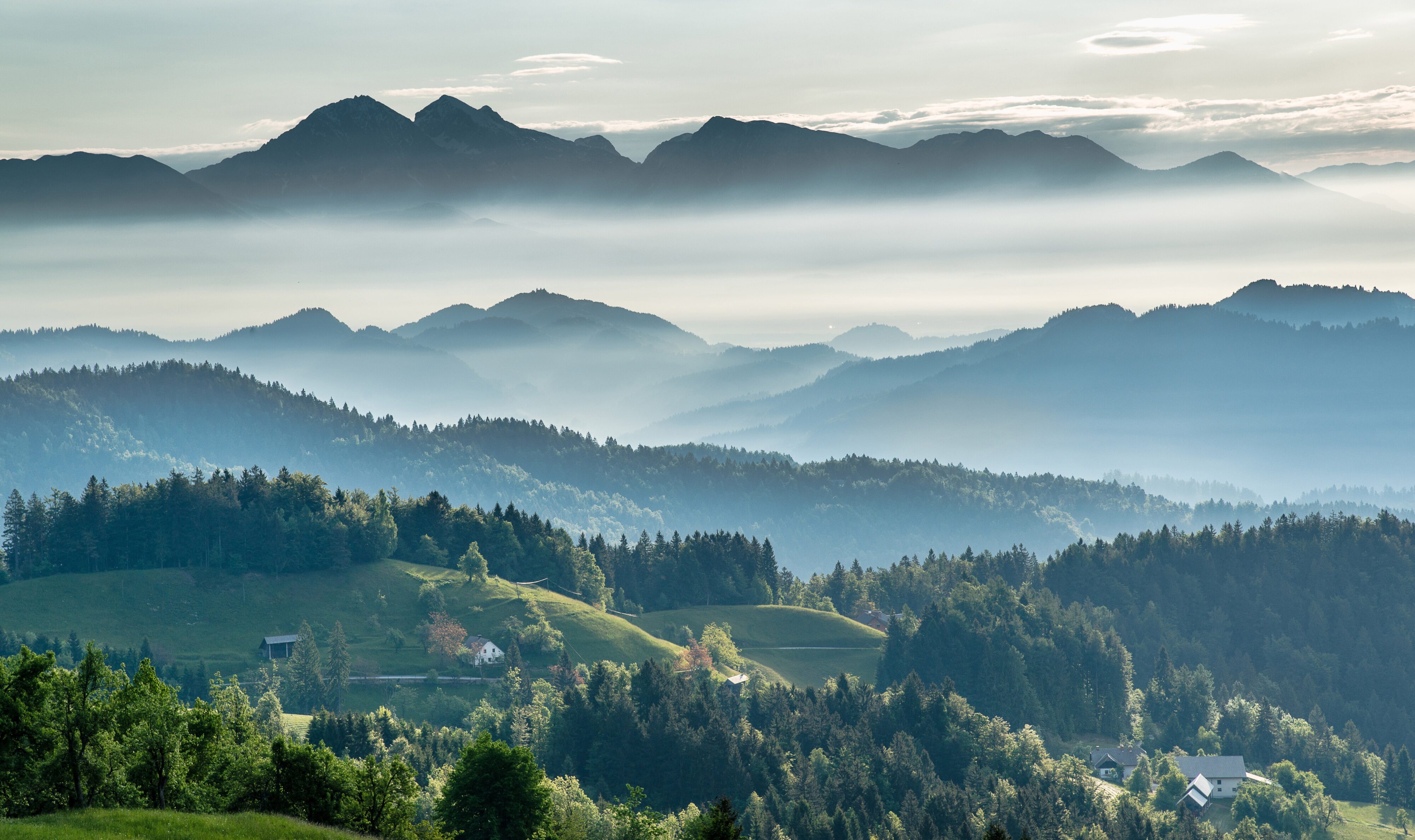
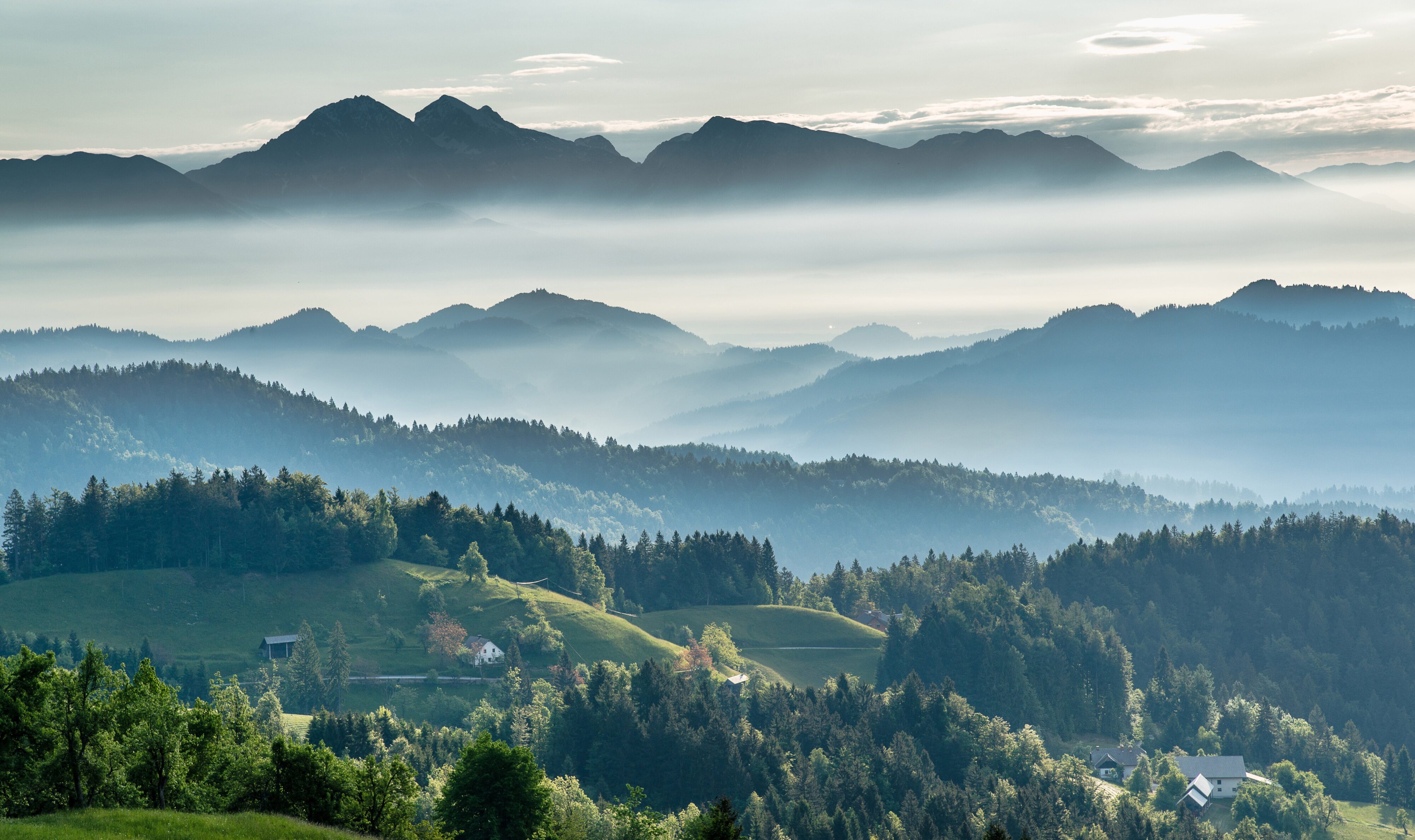
In the previous post, we looked at cloud-native relational databases. Next up, NoSQL databases.
NoSQL databases address a different set of problems compared to relational databases. They allow huge amounts of unstructured data to be stored. There are multiple types of NoSQL databases: document, key-value, wide-column and graph, and each of these types has different strengths and covers different use-cases. Since each NoSQL database type supports a slightly different use-case, you need to research the databases beyond the best fit from a spatial perspective.
Spatial Support
AWS, Azure and Google all offer a NoSQL database, but only Microsoft has native geospatial support. Google Cloud Firestore offers support via a geohashing library.
Database | Geospatial Support | FME Support |
AWS Dynamo DB |
No support. There used to be support for geohashes via an official library, this project has now been archived. |
Read and write. |
Azure Cosmos DB Documentation | Type of support: Native Spatial types: Geography and Geometry Supported types: Points, Linestrings, Polygons, Multipolygons. Spatial Functions: Within, Distance, Intersects. |
Read, write and query. |
Google Cloud Firestore | Type of support: No native support, but there is support for geohashing via a 3rd party library. Spatial types: Geography Supported types: Points Spatial Functions: Within, Distance, Bearing |
– |
Only Cosmos DB supports the geometry data type, so if you want to use Google Cloud Firestore and your data lives on a cartesian plane, you will need to convert it. Also, unless you are using Cosmos DB, then there is only support for points. You also lose many spatial functions compared to a relational database. For example, on PostGIS, you have access to hundreds of spatial functions, and on Cosmos DB you have three: distance, intersects, and within. However, these functions are still extremely powerful as they can execute very quickly across billions of records.
Use Cases
With the rise of serverless relational databases, where do NoSQL databases fit in when applied to geospatial scenarios? Let’s first have a look at some of the key things NoSQL databases promise.
Performance
- Consistent, single-digit millisecond latency at any scale.
- Multi-region data replication with full read/write so you can get extremely fast local performance if your app user base is globally distributed.
Flexibility
- Flexible schemas mean you can adapt the table schema as your requirements change. This allows for seamless unstructured & semi-structured data ingestion.
- Serverless architecture means you can scale read/write capacity instantly.
NoSQL is really about performance at scale, below are two sample use-cases that produce large volumes of data with a geospatial component where I could see a NoSQL database being useful.
Mobile Application
A mobile app where the location of the user is a critical part of the app. The app only has several thousand users currently, but it might need to scale to millions of globally distributed users:
- If you are providing feedback to the user in the application based upon their location, then latency is critical as your location can change quickly.
- If you have billions of points of interest, being able to ask which is the closest one and get a response back almost instantly.
- If you need to track the location of millions of users and you need unconstrained read/write.
Storing Sensor Data
If you are reading data from a sensor (e.g. GPS position of a vehicle fleet), and you want to store the data before writing queries to extract subsets of the data into a data warehouse for more complex analysis.
- You need unconstrained write because there could be spikes in the number of sensors reporting.
- The table will store 120 days of data which will equate to billions of rows.
- You need flexible schema support as the incoming data contains a location in lat/lng, but the structure of that data might vary based upon the device the user connects with (e.g. Android, iPhone etc) and will likely change as new devices are released.
Conclusion
Cloud NoSQL databases have limited spatial support and as a result, only cover a narrow set of use-cases. If, however, you are running into scaling or performance issues on a relational database they might be worth a look.
A NoSQL database paired with a data warehouse can also be a very powerful model which we will explore in the next section.